With today's large language models (LLMs), building a proof-of-concept (POC) for a chatbot is relatively easy, but turning that into a production-ready system is far more challenging. Early prototypes often impress in demos but can behave unpredictably in real-world use. The AI hype led to an explosion of AI projects and investments that have yet to produce return on investment. In large enterprises nearly nine out of 10 senior decision-makers said they have gen AI pilot fatigue and are shifting their investments to projects that will improve business performance, according to a recent survey from NTT DATA.”
Another survey's data suggests that nearly a third of CIOs don’t know which AI POCs achieve exit criteria metrics. In one interview with IDC’s Saroff, it was mentioned that some POCs don’t have an objective way to measure their success.
To bridge this gap, our team at ChatGenie employs rigorous evaluation cycles (“Evals”) to systematically test and improve our multi-AI Agentic Workflow that generate chatbots responses before deployment. Evals, short for evaluations, are essentially structured test runs that measure a LLM App’s performance on defined tasks1. A robust Eval regimen is therefore indispensable for transitioning from a promising POC to a reliable production chatbot.
The Role of Evals in Moving from POC to Production
In an enterprise setting, evaluation and quality assurance are the linchpins of a successful AI deployment. LLM-based chatbots do not follow deterministic rules; their responses emerge from complex probabilistic models, making outcomes hard to predict. Simply relying on ad-hoc manual testing is insufficient to guarantee consistent quality across the myriad things users might ask. This is why our team instituted a formal Evals process. This provides clear metrics on whether an update has truly improved the system or not. As one industry expert noted, without a proper evaluation system in place, you can’t even tell if changes are making things better or worse2. By treating evaluation as a first-class concern (much like QA in traditional software engineering), ChatGenie ensures their chatbot meets the high standards expected in production environments. Evals enable data-driven confidence: before any new model or prompt update is deployed to end-users, it must prove itself on extensive internal benchmarks.
What exactly do these Evals look like?
The following sections outline ChatGenie’s internal Eval process, step by step. This process encompasses everything from designing test datasets, using an LLM as an automated judge, generating model responses, calculating metrics, to incorporating human review. By understanding each stage, we can see how all the pieces come together to harden the chatbot’s performance.
1. Test Dataset Generation
The first step is assembling a comprehensive test dataset that represents the variety of user inputs the chatbot will encounter. ChatGenie pulls together a dataset from multiple sources:
Real customer messages: The team mines logs of actual user queries and conversations received from their Facebook Pages to capture genuine usage patterns. These real examples ensure the evaluation covers the queries and wording that real users employ, including colloquialisms, common typos, and unexpected questions.

Synthetic queries from LLMs: To supplement real data, ChatGenie generates additional test questions and scenarios using LLMs. This helps cover edge cases and rare situations that might not appear often in customer logs. For instance, the team might prompt an LLM to come up with tricky or adversarial questions, or to vary existing questions in novel ways. Using an LLM to create synthetic benchmarks in this way is a known practice to expand test coverage when human-crafted examples are limited3.
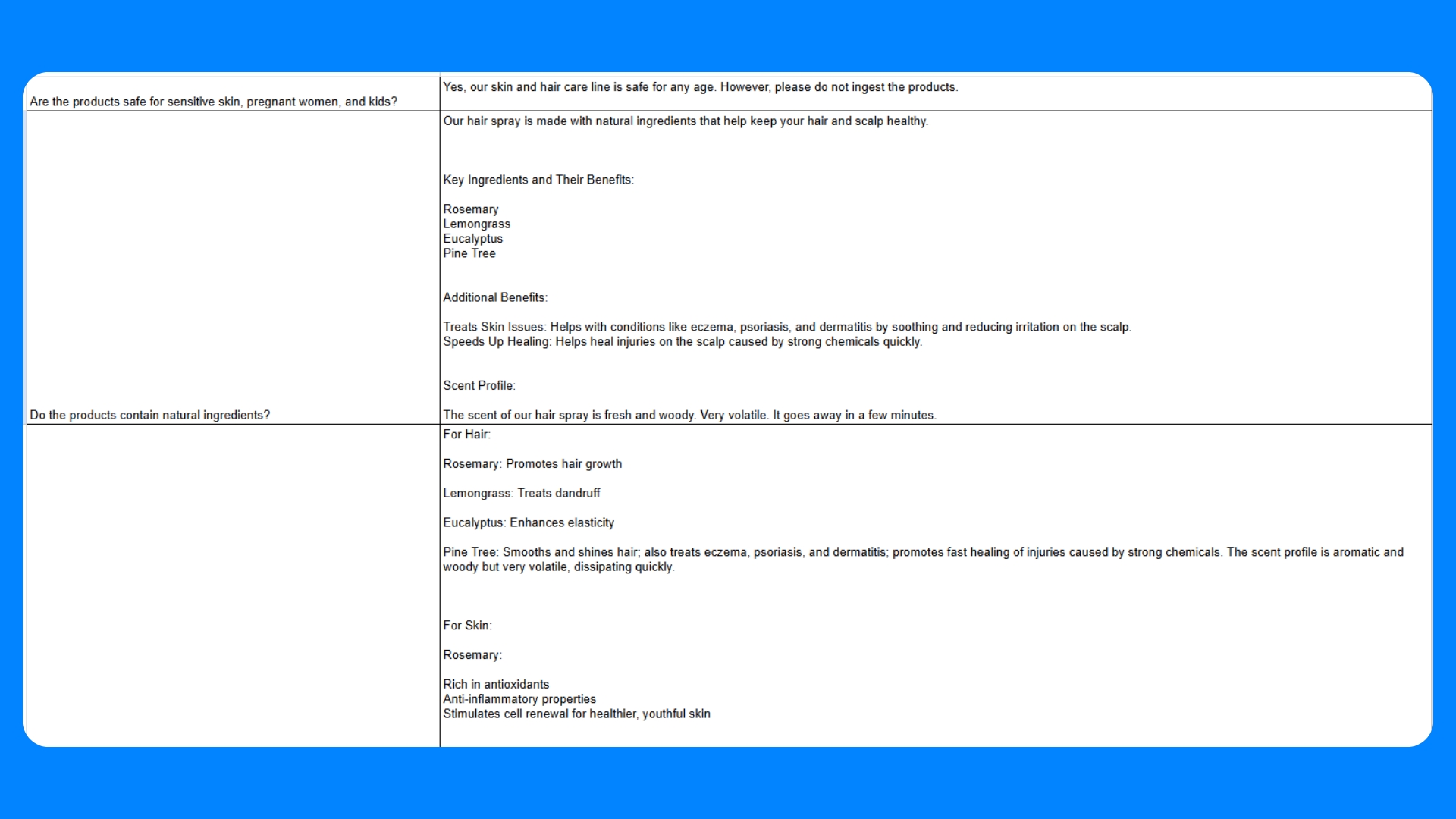
With a few shots prompting using the Deep research tool (OpenAI o3), it generated over 50 entries. In our attempts to generate synthetic data, it searched for existing brands within the same product category to have more context on what it will generate. From there, it also analyzed the scraped information from the website that we provided as reference.
2. Evaluation Method: LLM-as-a-Judge Setup
With a test dataset in hand, the next step is deciding how to evaluate the chatbot’s answers. Traditional metrics like exact match or BLEU don’t work well for conversational AI, since there are many ways to word a correct answer. ChatGenie chose an LLM-as-a-Judge approach for evaluation. In essence, this means using a separate AI (an LLM) to judge the quality of the chatbot’s responses. This method has gained popularity as a practical alternative to costly human evaluation for open-ended tasks4.
How does LLM-as-a-Judge work?
Our team employed a strong reference model (for example, GPT-4o or a similar top-tier LLM) and provides it with a carefully crafted evaluation prompt. This prompt instructs the judge model to analyze a given user query and the chatbot’s answer, and then determine if the answer is correct, helpful, and in line with expectations. The judge LLM will be asked to output a categorical verdict either “PASS” or “FAIL”. Importantly, the evaluation criteria are defined by ChatGenie’s requirements — for instance, correctness of information, completeness of the answer, and so on. By defining these criteria explicitly in the prompt, we ensure the judge LLM knows what “good” looks like in the context of the application.
Using an LLM in this role offers several benefits. First, it can handle the nuanced, context-rich nature of chatbot output evaluation: it can understand if a response is relevant and accurate without needing an exact match to a reference answer4. Second, it scales far better than human reviewers — the AI judge can evaluate thousands of responses quickly and consistently. (This consistency is valuable; unlike human evaluators who might have varying opinions, the LLM judge applies the same criteria uniformly.) Third, it’s flexible: we can easily adjust the evaluation prompt to focus on new aspects or fine-tune the strictness of the scoring. In summary, LLM-as-a-Judge provides an automated yet human-like evaluator for chatbot performance. It’s an approach increasingly seen in enterprise AI evaluations, where LLMs are used as unbiased, tireless judges of conversational quality4[^18].
To implement this, we set up the evaluation pipeline such that for each test query, after the chatbot produces an answer (in the next step), the judge model is invoked with the prompt containing that query and answer. The output from the judge is recorded for analysis.
3. Generate ChatGenie Chatbot Output
Once the evaluation mechanism is ready, we execute the chatbot agentic workflow on the entire test dataset. This step is essentially running the chatbot in a controlled batch mode to gather its responses for each test query:
- The latest model version of agentic worklow (with all current tweaks, prompts, and knowledge base if applicable) is used. This ensures the evaluation reflects exactly what would happen if this version went live. Consistency here is key: the same configuration (model parameters, temperature settings, system prompts, etc.) that is intended for production is applied during the eval run.
- Each query from the test set is fed into the chatbot as if it were a user message excluding customer profile memory, etc. This is automated evaluation that iterates over the list of test inputs and calls the chatbot agentic workflow each one.
- The chatbot’s response output for each query is recorded. On the Evaluation page, a tableview display will log the input, the chatbot’s answer, and confidence scores.
By automating response generation, we can very quickly simulate an entire mock chat session corpus. Instead of waiting for users to interact with the new version, they proactively generate outcomes on known challenges. This provides immediate insight into how the model will behave when confronted with the range of questions in the test set. It’s essentially a dry-run of the chatbot in the lab.
4. Running the Evaluation & Computing Metrics
With both the test inputs and the chatbot’s outputs in hand (from step 3), and the LLM judge set up (from step 2), we proceed to run the evaluation. In this stage, each Q&A pair is fed into the judge model, and the judge’s decisions are collected to quantify performance:
- Automated scoring: For each test case, the judge LLM evaluates the chatbot’s response. For example, the criterion is simply correctness, the judge will output "PASS"/"FAIL" which can be mapped to binary scores. Our current focus is primarily on whether the answer solved the user’s request appropriately, as this is the critical measure of success. The result is a list of judgments corresponding to all the responses.
- Accuracy calculation: Using the judge’s outputs, we compute the overall accuracy – essentially the percentage of test queries for which the chatbot’s answer was deemed correct. If out of 500 test questions, the judge marked 450 as correct, the accuracy would be 90%. This metric gives a top-level view of the chatbot's quality. It’s a straightforward measure: how often are we getting it right?
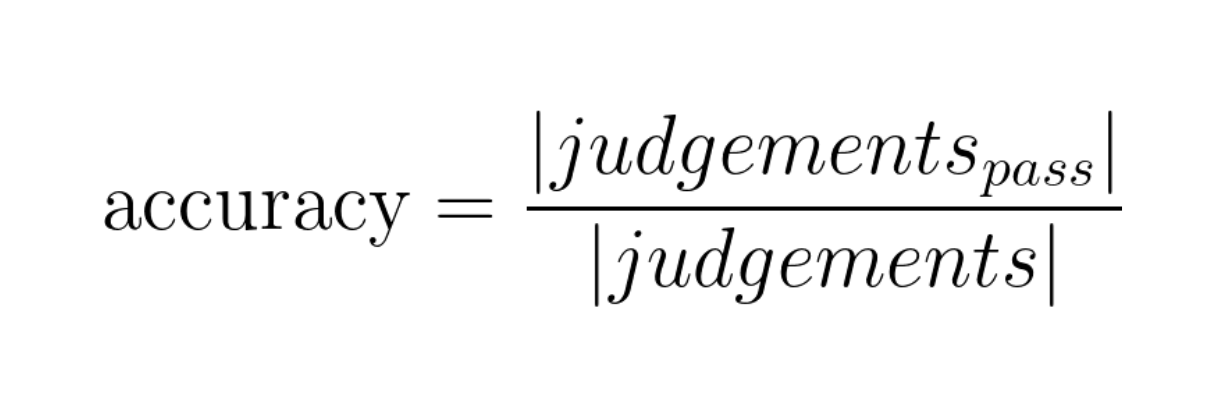
Weighted accuracy rate is the rebalanced accuracy rate based on the AI’s confidence in its judgement. Below is how the Weighted accuracy rate is computed on this simple formula:
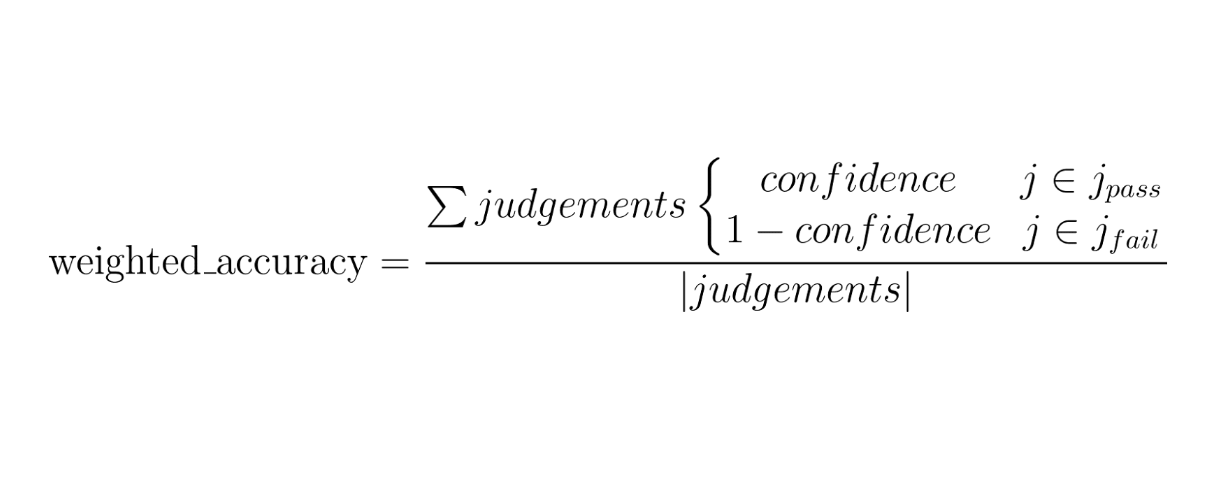
After running the eval, the results are compiled into a report for the team. Typically, this includes the overall accuracy, weighted accuracy, and any pertinent breakdowns or examples of failures. For instance, the team might see that the chatbot has 95% accuracy on general FAQs but only 70% on complex multi-turn requests – pinpointing an area for improvement.
In our first few runs, we saw an impressive 95.52% overall accuracy rate and 94.71% weighted accurate rate though there are few case where we saw false positives and negatives.
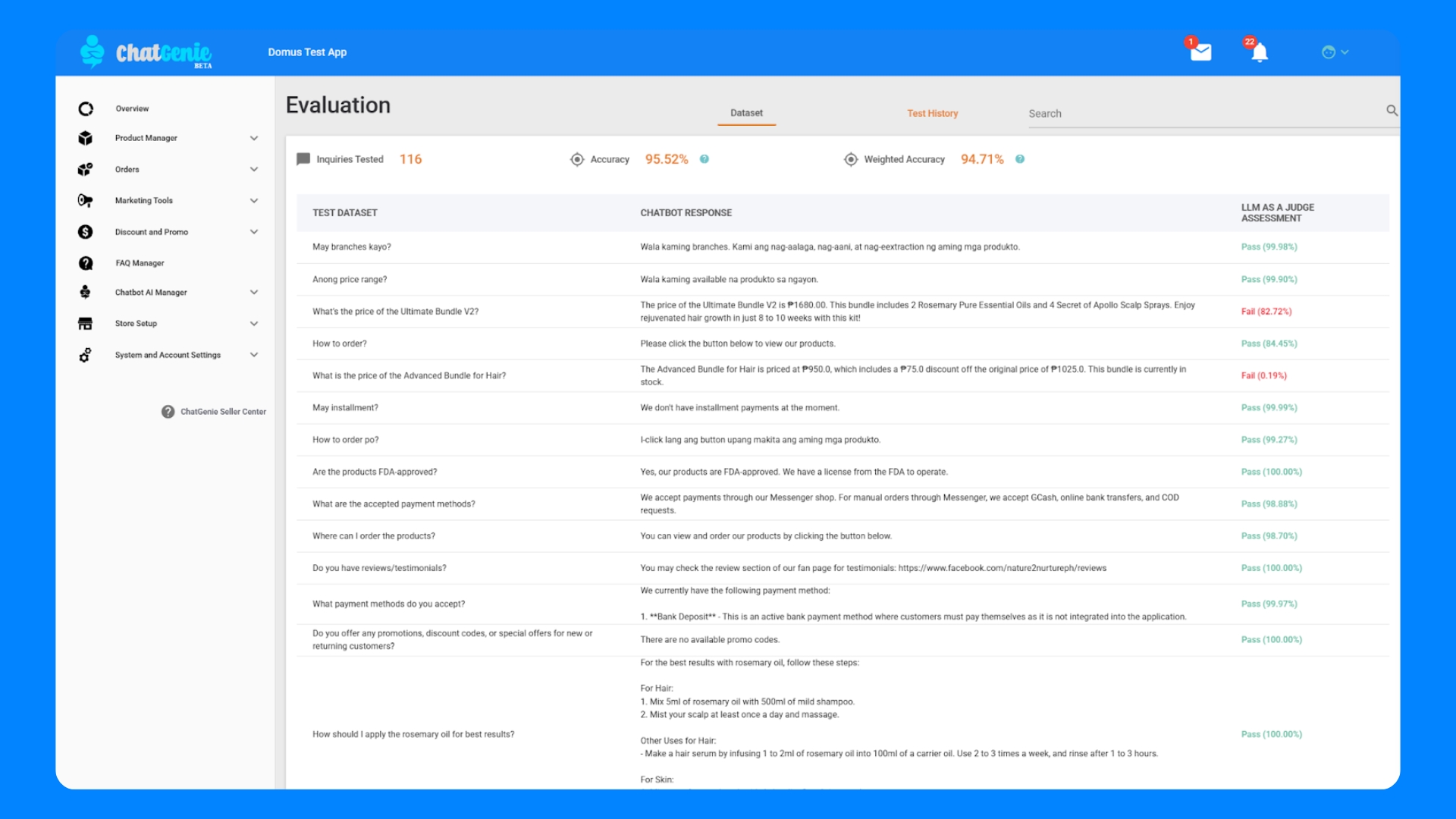
5. Manual Review and Iterative Improvement
Numbers and automated judgments alone don’t tell the whole story. In the final step of the Eval loop, human experts dive into the results to glean insights and make iterative improvements:
- Manual annotation and verification: Our team reviews a subset of the chatbot’s responses along with the LLM judge’s evaluation. Our human evaluation team pay special attention to the cases where the judge flagged an error. Here, the team manually checks if the judgment was correct – did the chatbot truly err, and if so, how? In some cases, they might find the chatbot’s answer was acceptable and the judge was overly harsh or misunderstood context (LLM judges, while very advanced, can occasionally mis-evaluate nuanced cases). The team can then refine the judge’s prompt or rules to handle such cases, or simply note them as limitations. Often, we annotate these cases with the correct evaluation (overriding the judge if necessary) to accumulate a set of human-verified examples. This helps in gradually improving the evaluation criteria and the test dataset for future runs.
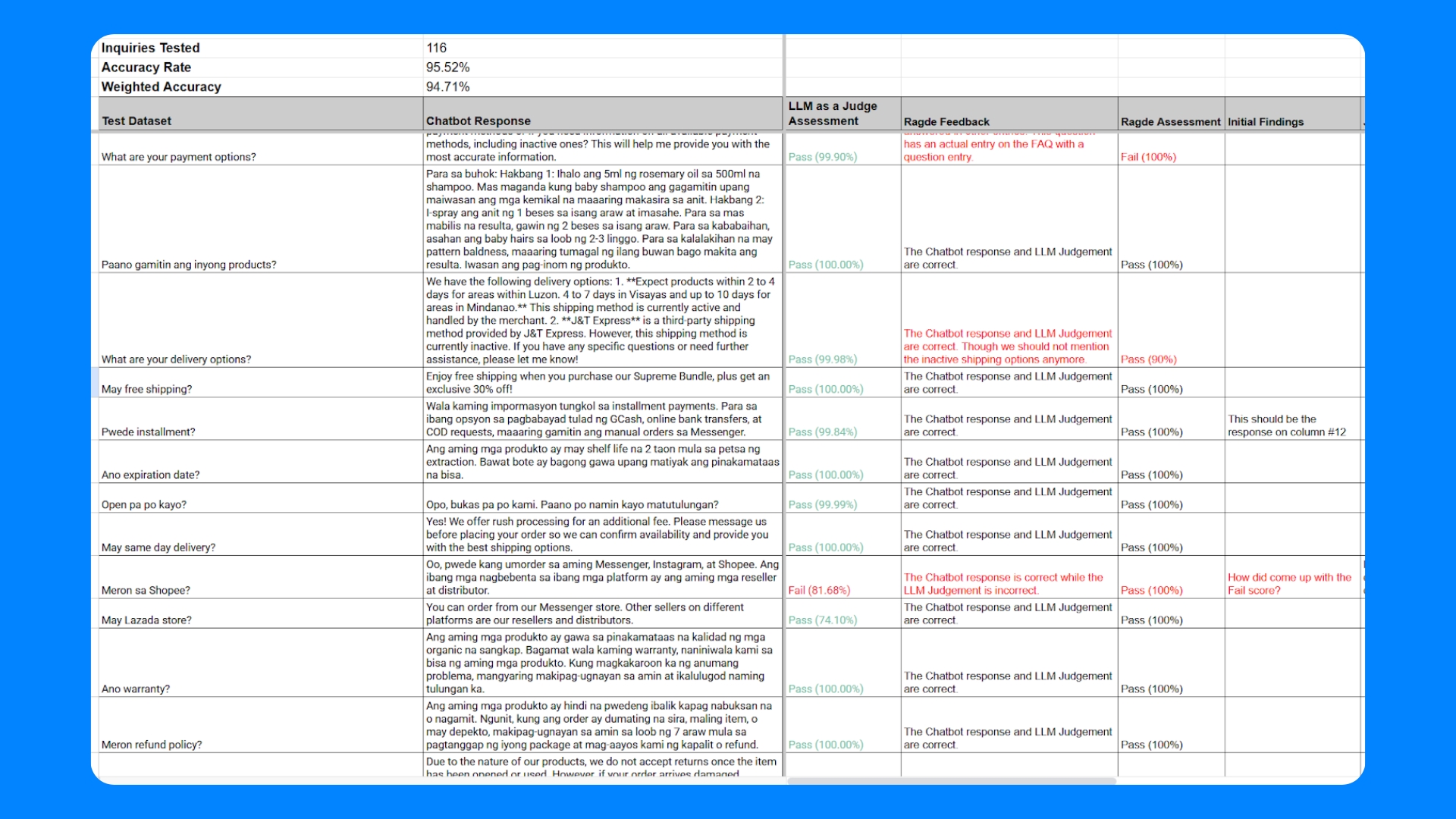
- Error analysis: For each confirmed failure of the chatbot, the team performs root-cause analysis. For example, if the chatbot gave an incorrect answer to a policy question, was it because it lacked information in its knowledge base? Or did it misunderstand the question? Or did it refuse when it should have answered? By categorizing the failure modes (e.g., factual error, refusal, formatting issue, etc.), we can decide on targeted fixes.
- Improvement actions: Based on the analysis, the team implements changes to the chatbot agentic workflow. This could involve many types of interventions: updating the prompt instructions, adjusting the retrieval mechanism (for a RAG system) to bring in relevant data, or even adding explicit rules/guardrails for certain scenarios. For instance, if Evals show the bot often fails at multi-turn clarifications, we might refine how conversation history is provided to the model. Each change is aimed at addressing one of the weaknesses revealed by the evaluation.
- Iterative cycle: After making improvements, our team doesn’t simply assume all is well – we always run the Evals again. The updated chatbot is put through the same paces with the test dataset, and new accuracy metrics are obtained. Ideally, the accuracy and weighted accuracy should improve, and specific categories of queries that were weak should show better results. If new issues appear (sometimes a fix in one area can surface a regression elsewhere), those will be caught by the Evals as well. This iterative loop continues until the chatbot reaches a performance level that meets the company's quality bar for production. In practice, ChatGenie might run through this cycle many times (design eval -> generate responses -> measure -> refine) during a development phase. Evals become a form of unit testing for the AI: every time something is changed, you run the tests to ensure nothing is broken and the overall performance is trending up.
Throughout this process, out team also keeps an eye on evaluation drift – if the judge LLM or criteria change, our team needs to calibrate to ensure that scores remain comparable over time. Keeping a history of evaluation results across versions helps in tracking progress. By the time the chatbot is ready to deploy, the team has high confidence (backed by metrics and validation examples) in how it will perform.
Conclusion: Evals as a gold standard to Deployment Success
Our methodical Evals process significantly raises the odds of a successful deployment. By catching errors and weaknesses early in a controlled setting, the team avoids nasty surprises in production. This rigorous evaluation practice means that when the chatbot finally interacts with real customers at scale, it has already been battle-tested against countless scenarios. In effect, Evals serve as a safety net and a quality filter — only chatbot versions that pass the eval benchmarks get the green light for release. The result is higher reliability, better user satisfaction, and fewer emergency rollbacks or hotfixes in production. Teams can deploy new updates with greater peace of mind, knowing that they've been vetted in a realistic simulation of user interactions.
Another advantage is faster iteration with confidence. Evals enable our team to practice evaluation-driven development, where every change is validated by targeted tests6. This approach is analogous to test-driven development in software engineering, and it’s emerging as a best practice for AI projects across the industry. Enterprises are recognizing that a well-defined eval pipeline is not just a nice-to-have, but a necessity when working with AI systems that learn and change. By standardizing such evaluation frameworks (similar to how unit tests are standard for code), organizations can ensure consistent quality and accountability for their AI deployments. Frameworks like OpenAI’s Evals1 and other tools are increasingly available to help teams implement these best practices uniformly.
In summary, our Evals process – from robust dataset generation to LLM-as-a-judge assessments and human-in-the-loop refinements – has been instrumental in turning a cool demo into a dependable enterprise solution. It highlights how rigorous evaluation is the key to unlocking production success. By championing a culture of continuous testing and improvement, our team not only delivers a high-performing chatbot for its users, but also sets a blueprint that other AI projects can follow to achieve similar enterprise-grade reliability.
Footnotes
- OpenAI, openai/evals (GitHub repository), 2024. (“Evals is a framework for evaluating LLMs and LLM systems, and an open-source registry of benchmarks.”) ↩ ↩2
- Christopher Robert, “Beyond POCs: Evaluation and QA for production-quality chatbots,” LinkedIn, Mar. 21, 2024. (Noting that without a good system for evaluation, one cannot discern forward vs. backward progress in chatbot improvements.) ↩ ↩2
- Hakan Tekgul, “LLM-as-a-Judge Evaluation for GenAI Use-Cases,” Arize AI blog, Nov. 11, 2024. (Describes using LLMs to create synthetic benchmarks for evaluating other LLMs, especially when human feedback is scarce, enabling valuable testing during development.) ↩
- Evidently AI, “LLM-as-a-Judge: a complete guide to using LLMs for evaluations,” Feb. 19, 2025. (LLM-as-a-Judge is an evaluation method that uses a language model with a dedicated prompt to rate another model’s output based on defined criteria, providing a flexible approximation of human judgment.) ↩ ↩2 ↩3
- Sumit Biswas, “Evaluating Large Language Models (LLMs): A Standard Set of Metrics for Accurate Assessment,” LinkedIn, 2023. (Discusses common evaluation metrics for LLMs, including accuracy and weighted accuracy, illustrating how weighted accuracy can account for differences in class frequencies or importance.) ↩
- Devin Stein, “Iterating Towards LLM Reliability with Evaluation Driven Development,” LangChain Blog, Mar. 11, 2024. (Introduces the concept of evaluation-driven development, emphasizing that continuously testing LLM outputs after each change allows teams to ship updates with confidence in their impact on reliability.) ↩